META-LEARNING: The Art of Learning to Learn
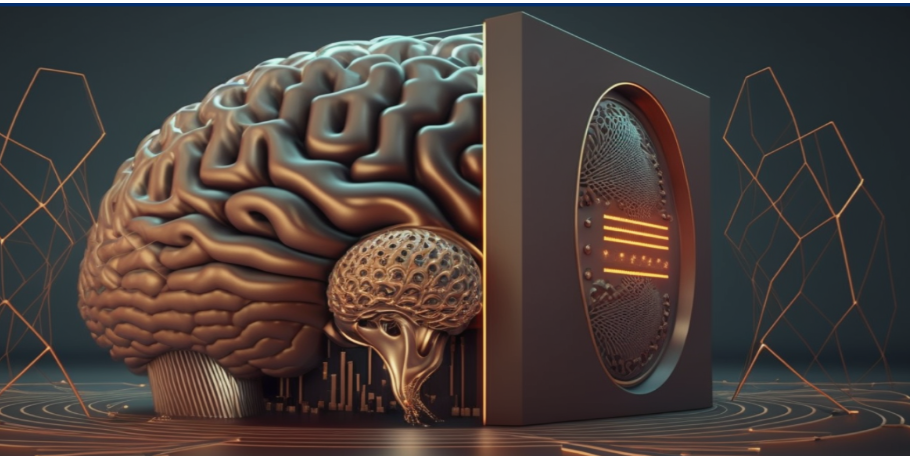
"Education is not the learning of facts, but the training of the mind to think."
- Albert Einstein
This quote by Albert Einstein emphasizes that education goes beyond the mere accumulation of facts and highlights the importance of developing a mindset focused on learning and critical thinking. It aligns with the concept of meta-learning, which focuses on training the mind to learn how to learn. It emphasizes that education is not simply about acquiring facts but rather developing the skills and abilities to adapt, generalize, and learn efficiently in various situations.
Understanding meta in Meta learning : The term "meta" in meta-learning refers to something being self-referential or higher-order. In the context of machine learning, it means learning about learning itself. Meta-learning focuses on training models to become better learners and adapt quickly to new tasks. So, meta-learning is about learning to optimize the learning process.
WHAT IS META-LEARNING ?
Meta-learning, also known as "learning to learn," is a concept that refers to teaching a machine learning model to learn how to learn new things quickly. It's like training a model to become a better learner itself.
Imagine you are a student who wants to excel in exams. You have observed that studying the same way is not effective, as different subjects require different techniques. Sometimes you need to read textbooks, solve practice problems and work on group projects. You realize that you need to learn how to learn and choose the most effective approach for each subject.
To become a better learner, you decide to employ meta-learning techniques. Here’s how it works:
1. Meta-Level: You begin by reflecting on your learning experiences across various subjects.You identify patterns such as which techniques work best for different types of subjects. This higher-level knowledge about effective learning strategies becomes your "meta-knowledge."
2. Task-Level: Now, when you encounter a new subject, you apply your meta-knowledge to adapt your study approach. For example, if it's a maths subject, you know that solving practice problems is crucial. If it's a social subject, you understand that reading and analysing texts is more important. By quickly adapting your study methods based on the subject, you optimize your learning process.
Through meta-learning, you have learned how to learn effectively. You have gained insights to become adept at choosing the most appropriate approach for each new topic or subject.
META-LEARNING VS TRADITIONAL MACHINE LEARNING :
In machine learning, a model is trained on a specific task using a dataset. Once trained, the model makes predictions based on that training. However, it may struggle when faced with a new task or a situation it hasn't come across.
Meta-learning addresses this limitation by training models on multiple related tasks. The model learns common patterns and strategies which enables it to generalize and adapt quickly to new, unseen tasks. It learns how to extract relevant information, identify important features, and make accurate predictions even with limited data.
WHY INTEREST IN META-LEARNING ?
Meta-learning offers unique advantages and solutions to specific issues faced by machine learning algorithms. Let’s address some key points:
- Handling limited data: It enables models to learn quickly by incorporating previous knowledge and experience.
- Adaptability to new tasks: It improves the ability of models to generalize and adapt to new tasks or situations.
- Optimization and Hyperparameter tuning: Meta-learning recommends suitable hyperparameters and optimization strategies for different tasks. It optimizes the learning process itself, improving efficiency.
While the machine learning techniques remain valuable, meta-learning equips them with the ability to learn how to learn, making it a valuable addition.
WORKING OF META-LEARNING ALGORITHMS :
Meta-learning algorithms aim to teach models how to learn new tasks and adapt quickly to new situations. Let’s see a step-by-step explanation of how meta-learning algorithms work:
Step-1: Training on base tasks
Meta-learning starts by training a model on a set of base tasks. Each base task consists of a training dataset and a specific objective or prediction to be achieved.
Step-2: Meta-training
Once the model has learned from the base tasks, it moves to the meta-training phase. During meta-training, the model learns to generalize from the knowledge gained in the base tasks and apply it to new tasks.
Step-3: Meta-testing
After meta-training, the model is tested on new tasks that it hasn't encountered before. The goal is to assess how well the model generalizes and adapts to these new tasks with limited data or examples.
Step-4: Iteration and improvement
The meta-learning process is iterative. The model continuously learns from new tasks, further improving its ability to learn and adapt. It improves its performance with each iteration.
The key idea behind meta-learning algorithms is to extract common patterns and strategies from base tasks, enabling the model to learn quickly and effectively on new tasks with limited data.
REAL-WORLD APPLICATIONS :
Meta-learning with its focus on teaching has found applications in various domains:
- Few-Shot Learning: Meta-learning algorithms excel in scenarios where there is limited labelled data available for each class. By leveraging prior knowledge from similar tasks, meta-learning enables models to quickly adapt and recognize new concepts with only a few examples. This is beneficial in areas such as image classification and object detection.
- Hyperparameter Optimization: Meta-learning can aid in automating the process of hyperparameter tuning. By training models on a variety of tasks with different hyperparameters, meta-learning algorithms learn to recommend suitable hyperparameter settings for new tasks. This improves the efficiency and performance of the models.
- Transfer Learning: Meta-learning enables effective transfer of knowledge from one task to another. By leveraging prior knowledge and experience from related tasks, models can generalize and apply their learned knowledge to new, unseen tasks. This is valuable in applications such as natural language processing.
- Domain Adaptation: Meta-learning facilitates knowledge transfer across domains. By training models on diverse tasks from different domains, they learn to adapt and generalize their knowledge to new, unseen domains. This is particularly useful when dealing with limited labelled data in target domains, such as in computer vision.
These are just a few examples of how meta-learning is applied in real-world scenarios.
CONCLUSION :
Meta-learning offers significant potential in the field of machine learning by teaching models how to learn and adapt quickly to new tasks. The applications of meta-learning are vast and diverse. From few-shot learning to reinforcement learning, meta-learning algorithms offer practical solutions to address real-world challenges. As research and advancements in meta-learning continue, we can experience its impact in real-world applications, driving the development of more capable and flexible learning systems.
REFERENCES :
- https://machinelearningmastery.com/meta-learning-in-machine-learning/
- https://www.analyticsvidhya.com/blog/2022/09/meta-learning-structure-advantages-examples/
- https://medium.com/abacus-ai/a-beginners-guide-to-meta-learning-73bb027007a
- https://www.improvestudyhabits.com/exploring-the-world-of-meta-learning-what-it-is-how-it-works-and-its-many-applications/
- https://research.aimultiple.com/meta-learning/
ALSO CHECKOUT :
- Curious about the different roles and career paths in data science, refer to this link for an in-depth exploration.
- Want to explore more such insightful articles then don’t forget to check this link.
- Check this link to explore about a testing company.
By Soumya G